Today's fast-paced laboratory environments generate sheervolumes of data that can feel like a tidal wave. From sophisticated analyticalinstruments to meticulously recorded experiments, information pours in fromevery direction. The key to successfully surfing this data tsunami lies in thestrategic implementation of robust systems and platforms for laboratory datamanagement.
In this blog post, we’ll look at the historical role of Scientific Data Management Systems (SDMS), explore the diverse landscape of data sources within the lab, and highlight the power of modern scientific data platforms for revolutionizing how we manage, analyze, and ultimately, leverage our valuable scientific data.
Understanding the Traditional Scientific Data Management System (SDMS)
Standalone SDMS solutions were developed to address historical business concerns, particularly the need for additional storage capacity to manage growing data volumes. These systems excel at capturing, cataloging, archiving, and retrieving data, making them ideal for laboratories focused on building a strong data repository for inactive data. Examples of traditional standalone solutions include Waters NuGenesis, Agaram Technologies’ Logilab, and Agilent Open Lab.
Key features and benefits:
- Instrument integration(focus on direct capture)
- Centralized storage and organization
- Basic search and retrieval capabilities
- Compliance features (audit trails, security)
- Manage instrument data(chromatography, spectroscopy, etc.) and LIMS/ELN data
Challenges:
- Often siloed and difficult to integrate with other systems (e.g., an enterprise resource planning system)
- Limited analytical and visualization capabilities
- Poorly designed user interfaces
- Challenges in handling unstructured or novel data types
- May lack flexibility for modern data science workflows
- May require IT input to resolve issues
- Compliance concerns around user authentication
- Such traditional systems, focused on passive data storage, have lost favor as technology has evolved.
SDMS as a Data Source: Bridging the Gap
An existing SDMS can beleveraged as a valuable data source for other analytical tools and platforms. Data-sourced SDMS solutions provide a centralized location tomanage, store, and archive data, often as an extension to existing laboratoryinformatics systems such as Laboratory Information Management Systems (LIMS) orElectronic Lab Notebooks (ELN). These systems streamline data access andtracking by collecting data from multiple sources, including instruments,equipment, and other software platforms. Notable vendors offering SDMS modules [MC1] alongside their LIMS solutions include LabVantage, STARLIMS, SampleManager, and Sapio Sciences.
Key features and benefits:
- Increasedinteroperability and data accessibility
- APIs(Application Programming Interfaces) as enablers
- Dataextraction and transformation processes (ETL).
- Allow the use of SDMS data with LIMS, ELNs, or business intelligence tools
- Maximize the value of existing investments
- Enable cross-system analysis and insights
- Modernize the data infrastructure
Challenges:
- Data format inconsistencies and need for harmonization
- Potential performance bottle necks during data extraction
- Maintaining data integrity across systems
As the uses of an SDMS evolved alongside technological improvements, software providers saw opportunities for streamlining the flow of data through organizations. This led to the development of scientific data platforms.
The Rise of the Scientific Data Platform: A Comprehensive Approach
A scientific data platform is a more comprehensive and integrated solution for managing, analyzing, and sharing scientific data. Data platforms represent the next evolution in SDMS solutions, offering not only the core functionalities of traditional systems but also advanced capabilities for digital preservation, data transformation, and long-term accessibility.
These platforms address the growing industry concern of ensuring that stored data remains usable and accessible over time, even as file formats and technologies evolve. Examples of leading data platforms include ZONTAL, Tetra Science, and Scitara.

Key features and benefits:
- Broad data integration: Connecting to diverse instruments, systems (LIMS, ELN), and data sources (including SDMS)
- Advanced analytics and visualization: Built-in tools or seamless integration with data science platforms (e.g., Python, R)
- Data curation and contextualization: Tools for cleaning, standardizing, and enriching data with metadata
- Scalability and flexibility: Cloud-native or adaptable architectures to handle growing data volumes and evolving needs
- Support for FAIR data principles: Facilitating findability, accessibility, interoperability, and reusability of data
- Breaking down data silos and fostering a data-driven culture
- Accelerating scientific discovery and innovation
- Improving data quality and reproducibility
- Enabling advanced analytics and machine learning applications
Challenges:
- User adoption—the value in such a radical change in workflows and processes can be difficult to sell to your team
- Infrastructure costs—implementing a comprehensive scientific data platform may require upfront investments in software licenses, hardware infrastructure (especially for on-premises solutions), data migration services ,and customization
- Data quality and governance—A substantial effort to clean and harmonize data prior to ingestion in the platform is required to make meaning after implementation
Key Differences Summaized: A Comparative Table
The differences between the three systems can be summarized in terms of their complexity, scalability, functions, scopes, and capabilities, along with the ability to integrate and their particular focus.
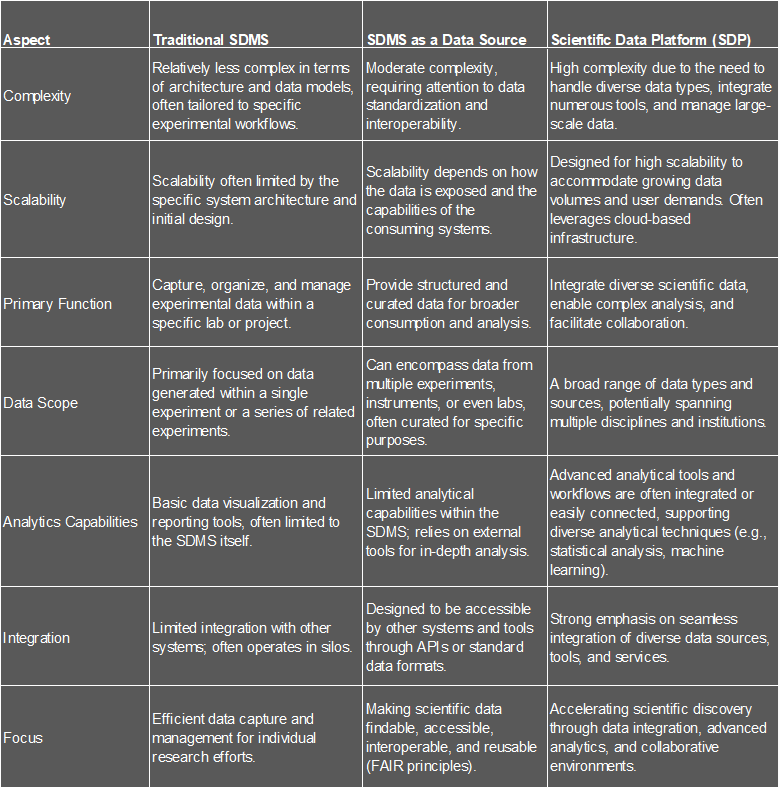
Choosing the Right Approach for Your Lab
Determining the best laboratory data management solution for your specific needs requires considering factors like:
- Current infrastructure and existing systems
- Data volume and complexity
- Analytical requirements
- Collaboration needs
- Budget and resources
- Long-term data strategy
When these are understood, it can be easier to use the table of key differences we’ve provided here to evaluate the pros and cons of each type of solution.
Embracing the Future of Scientific Data Management
Effective lab data management is more important than ever in modern laboratories. Without the right platform to stay on top of the data tsunami, laboratory professionals run the risk of being submerged in information before they can ever make meaning from it. An integrated, comprehensive scientific data platform can be much more efficient than the siloed data storage structures that existed a few short years ago.

An evaluation of your current data landscape is a worth while exercise that all labs should consider doing. This provides the opportunity to identify solutions that align with your future goals. CSols has the expertise to help you choose a solution and implement it successfully so that your lab staff will use it to the full extent of its capabilities.
Have you implemented a scientific data platform for more effective laboratory data management? Tell us some of your pro tips for successful adoption!
Comments