Today, it seems data analytics is being used everywhere. In this rapidly evolving business landscape, data analytics has emerged as a powerful tool for organizations to gain valuable insights, make informed decisions, and achieve strategic objectives. Data applications are no longer limited to just the realms of marketing and finance; they have permeated various sectors, including scientific research and laboratory management. Laboratories, whether in academia, healthcare, or industry, deal with copious amounts of data every day. The integration of data analytics tools can revolutionize how labs operate, leading to enhanced efficiency, better decision-making, and improved overall performance. The underlying reason for all this is the increased revenue that results.
There are thousands of articles about analytics indexed in Google right now; why should you read this specific blog post? As a lab informatics leader, CSols presents analytics from a life sciences point of view. So, dive into this topic with us for specific, actionable insights about how data analytics can be leveraged for your lab and upper management.
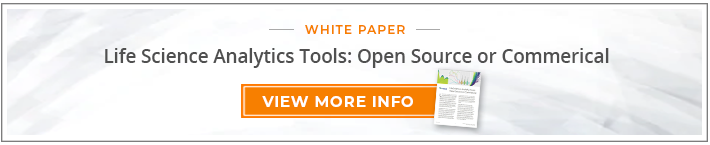
The Role of Data Analytics in Lab Management
Laboratories generate vast amounts of data from experiments, tests, and research activities. Traditionally, this data was stored in spreadsheets or paper records, making it challenging to extract meaningful insights or trends. Informatics and analytics tools help to efficiently collect, process, and analyze this data, enabling lab managers to optimize processes, troubleshoot issues, and make data-driven decisions. The following are a few practical applications for how labs can derive benefits from data analytics.

- Efficient Resource Allocation: Data analytics helps in identifying resource usage patterns, ensuring that equipment, materials, and personnel are allocated optimally. This prevents overstocking or underutilization, reducing operational costs.
- Quality Control and Assurance: By analyzing data from experiments and tests, labs can monitor the quality of results and detect anomalies early. This enhances the accuracy and reliability of outcomes, reducing the chances of errors or faulty conclusions.
- Predictive Maintenance: Data analytics tools can monitor equipment performance and usage patterns, enabling labs to schedule preventive maintenance before critical instruments fail during expensive campaign/batch runs (see Figure 1). This prevents costly downtime and promotes continuous operation.
- Workflow Optimization: Analytics can uncover bottlenecks or inefficiencies in laboratory processes. Lab managers can use this information to streamline workflows, minimize wait times, and enhance overall productivity.
- Regulatory Compliance: Many labs operate within strict regulatory frameworks. Adding data analytics can help to maintain compliance through accurate record-keeping, traceability, and adherence to standards. From our experience with many regulatory inspections, most inspectors will look very favorably on predictive tools to maintain compliance.

Implementing Data Analytics Tools in the Lab
The process of integrating data analytics tools into lab management involves the following key steps:
Data Collection and Storage
The foundation of effective data analytics is robust data collection and storage. Labs need to establish a structured and standardized method for collecting and storing data electronically. First steps here involve a Laboratory Information Management System (LIMS) or cloud-hosted databases. (CSols can help with choosing the right foundation.) Ensuring data accuracy and completeness is crucial at this stage.
Choosing the Right Analytics Tools
There is a wide array of available data analytics tools, ranging from simple spreadsheet software (e.g., Excel, Google Sheets, etc.) to complex statistical analysis software (e.g., PowerBI, SAS, Python, R, etc.). Selecting the right tools depends on the lab's specific needs and the complexity of the analyses required. Microsoft Excel can be suitable for initial stages, but as data volume and complexity increase, more advanced tools like Python, R, or dedicated analytics software may become necessary. It can be difficult to migrate from Excel to a more sophisticated tool while maintaining data integrity. If you expect your data capture needs to increase, a spreadsheet may not be the way to start.
Data Preprocessing and Cleaning
Raw data often requires preprocessing and cleaning before analysis. This involves removing duplicates, addressing missing values, and transforming data into a suitable format. Proper preprocessing ensures that the analytics process is based on accurate and reliable information. This is the transform stage in extract, transform, and load (ETL).
Exploratory Data Analysis (EDA)
EDA involves visually exploring data to identify patterns, trends, and outliers. This step helps in gaining initial insights into the data and formulating hypotheses for deeper analysis (e.g., variable weight, root cause, prediction, etc.). Devoting more time in this phase makes the next part, deriving insights, more enjoyable. Visualization tools like graphs, histograms, and scatter plots are essential for this stage. Whole companies and software titles have sprung up over the past decade to address this industry need (e.g., PowerBI, Tableau, Domo, etc.)
Advanced Analytics Techniques
Depending on the lab's objectives, specific advanced analytics techniques can be applied. These may include regression analysis, clustering (as in Figure 1), root cause and time-series analysis, among many others. These techniques provide deeper insights, allowing lab managers to make better informed decisions or to aggregate in a report for upper management.
Interpretation and Decision Making
The insights derived from data analytics need to be translated into actionable decisions. Lab managers should collaborate with domain experts (i.e., data engineers/scientists) to interpret the findings and determine the best course of action. This could involve adjusting experimental parameters, reallocating resources, or optimizing processes.

Leveraging Data Analytics for Upper Management
The benefits of data analytics extend beyond the laboratory itself. They can contribute to long-term strategic planning by providing insights into research trends, project success rates, and areas of innovation. Upper management can leverage these insights to help make holistic business process decisions that impact the entire organization in the following specific areas.
Performance Metrics and KPIs
Data analytics tools can provide upper management with real-time performance metrics and key performance indicators (KPIs) from the lab. This allows executives to monitor progress, identify trends, and assess the lab's contribution to organizational goals. These types of analytics are a perfect choice for presenting in a dashboard that updates in real-time.
Resource Allocation and Budgeting
Data-driven insights enable efficient resource allocation across different departments and projects. Budgets can be optimized based on actual data, leading to cost savings and improved financial planning.
As a specific example, Human Resource departments are finding success using predictive attrition modeling for balancing trade-offs between benefit/policy adjustments (e.g., health insurance, paternity leave, PTO, etc.) versus employee retention.
Strategic Planning
Upper management can make informed decisions about research direction, collaborations, and partnerships based on these insights.
As a specific example of strategic planning informed by data analytics, many delivery companies (FedEx, UPS, etc.) have implemented efficient route-planning algorithms that save millions of dollars annually to help them deliver goods from stop to stop. Imagine implementing such an algorithm for supply chains or production of a particularly expensive medicine. Process mapping can even help realize efficiencies within a specific production site if we’re talking about an analyst’s steps instead of delivery truck miles. In data science circles this classic optimization algorithm is referred to as the Traveling Salesman Problem (see Figure 2).
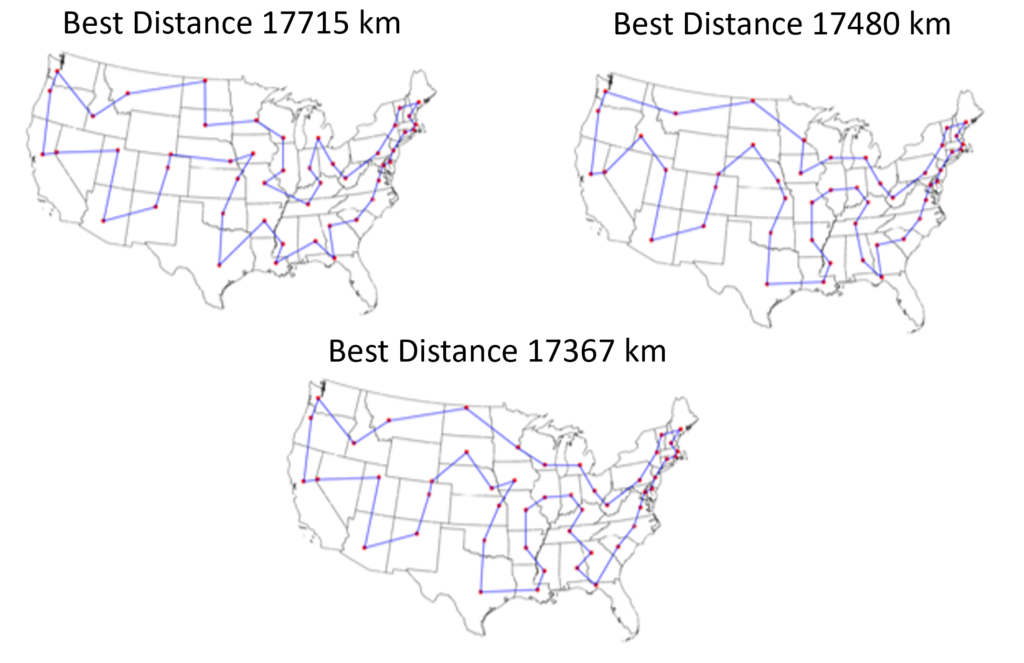
Regulatory Compliance and Risk Management
For labs operating in regulated industries, data analytics mitigates compliance risks from the application of standards and regulations. Upper management can monitor compliance metrics, identify potential risks early, and take proactive measures to moderate them. We’ve seen sites use analytics to categorize deviation types then trend occurrences to help sus out root causes. Regulatory agencies respond very favorably to this risk-based prevention.
Innovation and Growth Opportunities
By analyzing data from various experiments and projects, upper management can identify potential areas for innovation and growth. Data-driven insights may lead to the development of new products, services, or research directions.
As an example, many research labs are enjoying dramatic cost savings in the areas of bio and cheminformatics. What once was mostly guesswork, is now targeted statistically. Training machine learning and artificial intelligence to simulate likelihood of ligand binding in physiological systems can provide viable business models making it cost-effective to choose the next medicine to produce at scale.
Summary
The use of data analytics tools has the potential to revolutionize laboratory management. Labs that embrace data analytics can expect improved efficiency, quality, and decision-making capabilities. By following the steps outlined above and leveraging the power of data analytics, labs—and the broader organizations in which they operate—can unlock a new era of scientific discovery and organizational success. As technology continues to advance, the integration of data analytics in lab management is not just a luxury—it's a necessity for staying competitive and ensuring longevity in today's data.
How does your lab or upper management use data analytics? Tell us in the comments below.
Comments